This broad adoption by employees and organizations has introduced new cyber, technology and operational risks. These include the potential for social engineering, security breaches and systems failures. To properly assess and manage these risks, you must identify which AI technology you are going to look at.
Artificial intelligence is not a single, uniform technology but a broad category encompassing many different applications and techniques. To begin to understand AI risks, it is important to clearly define our terms before analyzing AI risk.
What is AI?
The first question we are often asked in relation to AI risk is “What do you mean by AI?”. AI, as it’s used in the media and more generally, is an umbrella term for many types of artificial intelligence applications and systems. These are often mixed together by people with different understandings or uses cases in mind. This can lead to confusion around what actual risks they face because the potential threats are not the same.
So I decided to ask some of the most common AI chatbots:
In a paragraph, describe what AI is and what are the different kinds based on capability most commonly used?
ChatGPT
Artificial Intelligence (AI) is the field of computer science that enables machines to mimic human intelligence, including learning, reasoning, and problem-solving. Based on capability, AI is classified into three types: Narrow AI, General AI, and Super AI. Narrow AI (Weak AI) is designed for specific tasks, such as virtual assistants, facial recognition, and recommendation systems. General AI (Strong AI) refers to machines with human-like intelligence that can perform any intellectual task a human can, though it remains theoretical. Super AI surpasses human intelligence, exhibiting advanced decision-making, creativity, and self-awareness, but it is purely hypothetical. These categories help define AI’s current applications and future possibilities.
Microsoft Copilot
Artificial Intelligence (AI) refers to the development of computer systems that can perform tasks typically requiring human intelligence, such as visual perception, speech recognition, decision-making, and language translation. Based on capability, AI can be categorized into three main types: Narrow AI, which is designed for specific tasks like virtual assistants (e.g., Siri, Alexa); General AI, which aims to perform any intellectual task that a human can do, though it remains largely theoretical at this stage; and Superintelligent AI, which surpasses human intelligence and capabilities, also a theoretical concept.
Claude
Artificial Intelligence (AI) refers to computer systems designed to perform tasks that typically require human intelligence, encompassing both narrow AI (designed for specific tasks) and general AI (hypothetically capable of human-like general intelligence). The most common types based on capability include machine learning systems that learn from data patterns, deep learning models using neural networks for complex pattern recognition, natural language processing systems for understanding and generating human language, computer vision systems for processing and analyzing visual information, expert systems that make decisions based on predefined rules, robotics AI for physical task automation, and reinforcement learning systems that learn through trial and error to optimize decision-making processes. While narrow AI excels in specific domains like image recognition, language translation, or game playing, artificial general intelligence (AGI) that matches or exceeds human-level cognition across all domains remains a theoretical goal.
Gemini
Artificial intelligence (AI) is the ability of a computer or machine to mimic human intelligence. This can involve things like learning, problem-solving, and decision-making. AI is a broad field, and there are many different types of AI. The most common types of AI are narrow or weak AI, general AI or strong AI, and super AI. Narrow AI is designed for a specific task, like playing chess or recommending products. It's the most common type of AI today. General AI is a theoretical type of AI with human-level intelligence, capable of performing any intellectual task a human can. Super AI is also theoretical and would surpass human intelligence in all aspects. While general and super AI are still largely in the realm of science fiction, narrow AI is already having a significant impact on our world.
The answers above are broadly similar. The most common type of AI technology used falls under narrow AI, sometimes called weak AI. This includes:
- Large Language Models (LLMs)
- Generative AI (GenAI)
The difference between LLMs and GenAI is their application. LLMs are a subset of generative AI and LLMs only generates text. GenAI applications can use various kinds of input, such as images or sounds as well as words, and the applications can generate content that includes text, images, sounds. Applications such as ChatGPT, Microsoft Copilot or Anthropic’s Claude are now called multimodal GenAI because they can process and generate more than just text.

Once you have identified the types of AI that you or your third parties are using and need to assess, you will be able to better scope the potential risks scenarios you and your company could face.
The CIA Triad and Common Risks Related to AI
For risk managers and policymakers, the CIA triad is useful for identifying common AI-related risks.

Confidentiality
- Employees using open source LLMs and leaking sensitive company information
- Third-party LLM has poor controls leading to your data being leaked via prompt injection
- Cyber criminals using AI tools to enhance attacks such as phishing which lead to a breach
Integrity
- Data poisoning of LLM leading to issues with the model outputs
- Training data used not properly secured or maintained leading to potential bias in its outputs
Availability
- Cyber criminals using LLM’s to discover and exploit zero day vulnerabilities
- Using LLMs for specific use cases and if unavailable leads to system outage
How do we analyze AI risk?
To help with identifying what the most relevant AI risk scenarios, we recommend using the steps outlined in the FAIR-AIR approach playbook. This approach helps risk analysts and risk leaders speak the same language as the business so that decision-makers and executives can understand the risk and what should be done to reduce the risk.
5 Steps to Analyze Risk Using the FAIR-AIR Approach
1) Contextualize
- Why are you analyzing this risk and what’s driving the reason behind it?
- What are vectors for AI Risk?
2) Scope
- Identify risk scenarios
- Identify the attack surface, threat actor, method of attack, and impact of threat on your asset
3) Quantify
- Determine Likelihood and Impact of the risk
4) Prioritize/Treat
- Determine Treatment options and prioritize those with the greatest impact
5) Decision-making
- Using results and treatment options decide on plan going forward
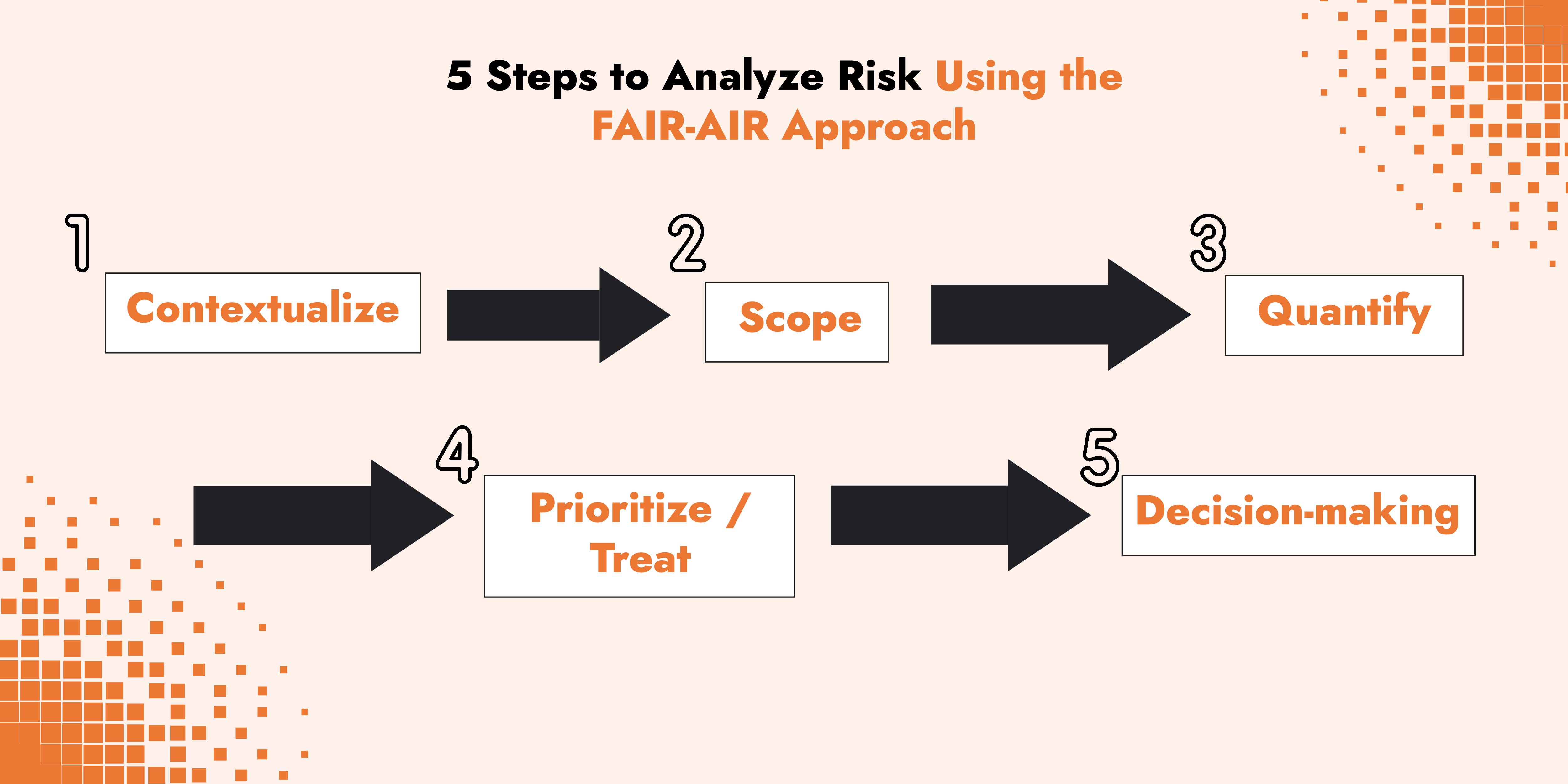
Ensure AI risk resilience with expert guidance
Schedule a call with one of our cyber and technology risk experts to learn more how we integrate open standards and frameworks to scope, assess, and manage your AI risks.
Example of an AI Risk Analysis Using FAIR-AIR
What is the risk of employees using open-source AI tools to generate reports by uploading sensitive client data?
By following the steps outlined by FAIR-AIR, you can build a data-driven picture of risk to better understand the potential risk, so that you can decide how to treat it.
We will use the example of a marketing agency with revenue of €200 million per year that has clients across the globe.
* Please note all figures and data listed are for illustrative purposes only.
1) Contextualize
We’re looking at this scenario as the company is worried about users uploading sensitive data and the lack of controls in place to prevent this. They are considering blocking access to unapproved tools.
2) Scope
- Asset: Sensitive customer and company data
- Threat Actor: Cyber criminals
- Attack Surface: Number of employees with access to the sensitive data
- Method of Attack: Model inversion attack
- Impact: Reputation damage leading to loss of existing and potential customer along with potential fines from customers and regulators
3) Quantify
- Likelihood: through research on usage of tools in the company, threat intelligence and external data points, there is a 1-7% likelihood, most likely 3%, of a data breach due to employees uploading sensitive customer data
- Impact: Estimated to be around €5 million in losses
4) Prioritize/Treat
The key risk driver is access to sensitive customer data so option include:
- Option 1: Block access to AI tools unless exception is approved (cost €100,000)
- Option 2: Improve User access controls to reduce number of users with access to sensitive data (cost €160,000)
- Option 3: Implement both options, 1 & 2 (cost €250,000)
5) Decision-making
- Both options are considered as equally likely to reduce the likelihood of this risk scenario occurring but improving access controls will have a higher cost. However, blocking access to AI tools is determined to have the negative side effect of reducing creativity amongst staff who use the tools, potentially leading to a loss of revenue. In this case, it’s agreed the control with the best Return on Investment (ROI) is option 2.
While AI is a new and rapidly evolving technology, as you can see in the example above the risks are similar to other existing technology when broken down using FAIR-AIR. Employees can upload or leak sensitive client data in multiple ways and using an open source tool like described is just an additional attack vector to consider.
The FAIR Institute published a series on their blog analyzing AI risk scenarios that address common threats. These demonstrate how to frame an incident so that you can measure the impact and likelihood of a threat event using a quantitative approach.
Data-Informed AI Opportunities
The adoption of AI tools and systems is accelerating, and with it comes a mix of opportunities and risks. A structured, data-driven approach helps organizations assess AI risks, quantify potential impact, and prioritize mitigation strategies. As seen in our example, AI risks aren’t entirely new and can resemble existing cybersecurity threats. The key is adapting risk management strategies to address AI’s unique attack vectors.
Rather than restricting AI use, organizations should take a risk-based approach, implementing controls that mitigate risks while still enabling employees to leverage AI’s benefits. Strong AI governance, clear policies, and continuous monitoring will be crucial to ensuring responsible AI adoption in an evolving threat landscape.
Don’t let unmanaged risks slow your innovation. C-Risk helps organizations take a data-driven, risk-based approach to AI adoption, ensuring security, compliance, and business continuity. Schedule a call with a C-Risk cyber and risk management expert to learn more about our business-centric and data-driven approach to risk management!
AI and Risk Analysis FAQ
What is a large language model or LLM?
A large language model (LLM) is a neural network, trained on huge amounts of mostly human-generated data. LLMs are built using deep learning techniques and can perform natural language processing tasks like answering questions, text generation and summarizing. The most popular LLMs that we interact with are GPTs or Generative Pre-trained Transformers, such as ChatGPT from OpenAI, Microsoft Copilot and Gemini from Google.
What is FAIR-AIR?
FAIR-AIR is an AI risk assessment approach designed to help risk analysts and leadership identify AI-related risks and make informed decisions on how to manage them. Developed by the GenAI workgroup of the FAIR Institute, it is based on the Open FAIR™ model for risk analysis. FAIR-AIR applies the FAIR framework’s techniques to model and quantify AI-related risks in probabilistic and financial terms.
We build scalable solutions to quantify cyber risk in financial terms so organizations can make informed decisions to improve governance and resilience.